Model Design, Development and Management
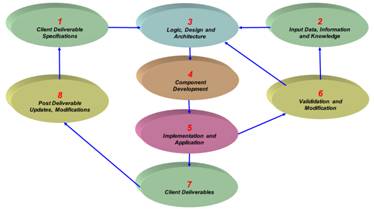
For clients that need to establish or improve their own in-house forecast and market modeling capabilities.
Our success is based on an Approach and rigorous methodology that guides us through the design, development and operational phases of building and implementing forecast models in a collaborative environment. This unique approach ensures that we avoid the mistakes and errors that others typically make. Our methodology is not packaged as an application, but rather manifests itself through our expert advice and counsel. We measure success as much by the increased understanding that the client accrues via the development process, as we do from the actual results produced by the models themselves.
Five broad areas encapsulate our approach and methodology:
- Decisions and Scope
- The Natural Process
- Taxonomies and Metrics
- Topology and Architecture
- Utility and Constraints
Decisions and Scope - What decisions will the model influence? This is the first question we ask since the answer defines the boundaries of the model and specifies the supporting research. Whether the objective is to build or validate business/marketing plans, acquire or divest a business, enter or exit a market, or allocate and marshal resources, the nature of the decisions will determine the model scope, scale and architecture.
One the most common forecasting mistakes is to confuse the company’s offering with the market. This often happens when clients fail to recognize that the introduction of emerging technologies is a migratory event. All technology enabled products or services are simply new ways of doing old things. Sometimes it is not clear that this is the case. Technology never changes basic human wants and needs; it just provides a better way of meeting them.
Successful technologies confer benefits and advantages on those that adopt them. It is critical that the model development effort consider not just the apparent market space, but the real alternatives and substitutes that compete. This requires discovery and understanding of the Natural Process governing individual and enterprise buying decisions and is particularly important when building forecast models for convergence products and services.
The Natural Process - Is the process that created the past the same process that will create the future? This is the single most important issue that needs to be addressed. Failure to ask this question (or worse, to merely assume that the answer is “Yes”) is the most common cause of a model’s failure to meet predictive success. Our recent financial crisis is a prima facie example. Many real world systems are stochastic, even chaotic, yet appear to be both well behaved and linear. Correlation does not imply causality; therefore, care must be taken when assuming that the future will simply be a continuation of the past. Causality must always be an input to the model, never a conclusion drawn from it.
Technology market models are inherently models of migration. The first modern electronic computing machines were replacements for manual or mechanical devices for pure computing and then commercial transactions. The first PCs were just a better way for people to write, perform arithmetic and organize data, replacing paper and pencil. More recently, technologies have provided users with better ways to communicate and access knowledge. In all cases, there was a prior individual or commercial behavior process utilizing a product or service whose users migrated to newer alternatives. Now we are on the verge of new migrations as Life Management behaviors are migrating to convergence products and services.
Forecasts predict the aggregate behavior of individual or enterprise buying agents. Even for highly defined and confined markets, it is prudent to ask the process question and reaffirm the assumptions concerning market behavior. Failure to do so will often manifest itself in an unpleasant surprise.
Taxonomies and Metrics - What are we measuring? What do we know? These are the next two questions in the design phase, and are highly dependent on the decisions set, and the assumptions about process.
"What are we measuring?" specifies the output metrics of the model. Models can be Demand-Consumption (measuring spending) or Supply-Sales (measuring revenues). Revenue models are inherently more complex in multi-tiered distribution markets requiring channel uplift adjustments. Multi-national models, whether spending or revenue, need to account for currency fluctuation. In addition to revenues or spending as measured in dollars, the model may include units and price per unit as output metrics. Many models, especially those with high migratory attributes, will include or be centrally driven by an installed base component that accounts for inertia in the market. In many cases the best metric that addresses the decisions of the client may be Market Share or Growth Rates.
“What do we know?” specifies the inputs to the model and perhaps more importantly, what data is missing and needs to be acquired. The input data, output metrics, and functional form of the model are interdependent and influence the choice of model architecture. However, if the elected model architecture requires data that is not currently available, then either a different architecture needs to be employed or the required data obtained. The difficulties in tailoring taxonomies to the model requirements need to be understood and addressed early in the planning phase.
The model dimensions and resulting taxonomies should be clear from the preliminary analysis concerning decisions, process and metrics. However, it is rare that all of the input data share a common vocabulary and structure. The input data may be freely available or purchased from many sources and include consumer and business demographic data, market and industry reports and databases, as well as primary research supporting the project. Daniel Research Group is especially proficient at merging, melding and manipulating these disparate data sets into a cohesive whole which is optimized for the clients’ model. We have developed solutions for taxonomy transformation, extrapolation and “tiling the plane” problems.
Topology and Architecture - What is the F in y = F(x)? Creating the algorithmic relationships between the input data and the output metric is the art of modeling. Our goal is to build models that are optimal for the following desirable attributes:
- Accuracy – predictions are correct.
- Adaptability – easily modified and expanded to meet changing real world conditions and client needs, with minimum additional time or budget investment.
- Viability – maintains its validity over its expected life and beyond.
- Intuitiveness – easy for analysts to use and generate immediate updated output.
In order to optimize for these attributes Daniel Research Groups builds models based on three design principles:
- Common Components
- Order of Resolution
- Modularity
Our clients’ models are constructed using Excel, Access and VBA. While some of our algorithms are proprietary, the visible and accessible elements of the model will be familiar to our clients’ analysts who are tasked with their use and will be easily supported by the clients’ own IT departments.
Order of Resolution is central to our design approach. The path from input to output is divided into discrete steps, each one defined in terms of the computational tasks that are required. Some of these steps may be functionally related with clear prerequisites or even recursive. Regardless of the algorithmic topology of the model, the computational topology will be linear.
The decision as to the order of these steps is critical to the optimization goal. Some general rules can be applied such as progressing from what you know best to what you know least. However, other factors including dependencies, minimizing files sizes, production run time, maintaining flexibility and adhering to the analysts’ worldview, may dictate a different order. Our experience, knowledge and skill in specifying the Order of Resolution is a core competency of Daniel Research Group.
Once the Order of Resolution is set, the final computational structure of the model can be determined. The steps are encapsulated in various Excel and Access components in a modular fashion that facilitates verification and validation at each step, ad hoc modification and expansion and efficient production performance.
Daniel Research Group has developed an inventory of approaches and methods that can be used in the construction of client models. We are particularly adept in the area of segmenting having developed proprietary techniques (GrowthSolver, SegmentSolver, MatrixSolver). These techniques are used to allocate top line forecasts to single or multiple segmenting taxonomies with maximum control over the trend and shape of all metrics, including growth curves. While the direction of most models is Top-Down, and some are Bottom-up. We have extensive expertise that supports development of models that are simultaneously Top-Down and Bottom-Up.
Utility and Constraints - Do I need to change the way I do my work? No. While our model building approach is structured, it supports an extraordinarily high degree of flexibility. We adapt our models to the worldviews of our clients by paying particular attention to the tools, methods and processes that our clients and their analysts are familiar with. In many cases, we integrate elements of the clients’ existing models, forecast applications and tracking systems into the models we build for them.
We also pay particular attention to validation and output presentation and understand the difficulty that an analyst might have in noticing data anomalies and other errors in a sea of numbers. Our models include both computational and graphical features and capabilities that allow for rapid identification and resolutions of these types of issues.
When engaged by a client to build a forecast model we recognize that there are several constraining variables including budget, urgency, frequency and timeliness of updates, as well as assimilation into their analytical practice. We take all of these into consideration when proposing a design and development approach and work with our clients in appropriating the best methodologies to meet their needs within these constraints.